Creating A New CNN¶
Hi! Welcome to \(\texttt{stella}\), a package to identify stellar flares using \(\textit{TESS}\) two-minute data. Here, we’ll run through an example of how to create a convolutional neural network (CNN) model and how to use it to predict where flares are in your own light curves. Let’s get started!
[3]:
import os, sys
sys.path.insert(1, '/Users/arcticfox/Documents/GitHub/stella/')
import stella
import numpy as np
from tqdm import tqdm_notebook
import matplotlib.pyplot as plt
plt.rcParams['font.size'] = 20
1.1 The Training Set¶
For this network, we’ll be using the flare catalog presented in Günther et al. (2020), which were identified and hand-labeled using all stars observed at two-minute cadence in \(\textit{TESS}\) Sectors 1 and 2. The catalog and the light curves can be downloaded through \(\texttt{stella}\) with the following:
[4]:
download = stella.DownloadSets(fn_dir='.')
download.download_catalog()
WARNING: AstropyDeprecationWarning: ./Guenther_2020_flare_catalog.txt already exists. Automatically overwriting ASCII files is deprecated. Use the argument 'overwrite=True' in the future. [astropy.io.ascii.ui]
WARNING: Logging before flag parsing goes to stderr.
W0714 08:45:08.602910 4409996736 logger.py:204] AstropyDeprecationWarning: ./Guenther_2020_flare_catalog.txt already exists. Automatically overwriting ASCII files is deprecated. Use the argument 'overwrite=True' in the future.
Et voila! A table of flares. For this demo, we’ll only be using a subset of targets. Please ignore this when creating your own CNN!!
And we’ll download that subset of light curves.
[5]:
download.flare_table = download.flare_table[0:100]
download.download_lightcurves()
0%| | 0/5 [00:00<?, ?it/s]//anaconda3/lib/python3.7/site-packages/lightkurve/lightcurvefile.py:47: LightkurveWarning: `LightCurveFile.header` is deprecated, please use `LightCurveFile.get_header()` instead.
LightkurveWarning)
20%|██ | 1/5 [00:08<00:32, 8.23s/it]//anaconda3/lib/python3.7/site-packages/lightkurve/lightcurvefile.py:47: LightkurveWarning: `LightCurveFile.header` is deprecated, please use `LightCurveFile.get_header()` instead.
LightkurveWarning)
40%|████ | 2/5 [00:18<00:26, 8.90s/it]//anaconda3/lib/python3.7/site-packages/lightkurve/lightcurvefile.py:47: LightkurveWarning: `LightCurveFile.header` is deprecated, please use `LightCurveFile.get_header()` instead.
LightkurveWarning)
60%|██████ | 3/5 [00:27<00:17, 8.87s/it]//anaconda3/lib/python3.7/site-packages/lightkurve/lightcurvefile.py:47: LightkurveWarning: `LightCurveFile.header` is deprecated, please use `LightCurveFile.get_header()` instead.
LightkurveWarning)
80%|████████ | 4/5 [00:36<00:08, 8.79s/it]//anaconda3/lib/python3.7/site-packages/lightkurve/lightcurvefile.py:47: LightkurveWarning: `LightCurveFile.header` is deprecated, please use `LightCurveFile.get_header()` instead.
LightkurveWarning)
100%|██████████| 5/5 [00:47<00:00, 9.53s/it]
These light curve files are downloaded to the preset fn_dir
set. The light curves are downloaded through \(\texttt{lightkurve}\). They are then reformatted to .npy
files to save space and the original FITS files are deleted. If you wish to keep the original FITS files, you can set download.download_lightcurves(remove_fits=False)
.
First, we need to do a bit of pre-processing of our light curves. The details of this can be found in Feinstein et al. (submitted). The pre-processing is necessary to reformat the light curves such that the Tensorflow modules work. The recommended settings (such as the length of light curve fed into the neural network and the fractional balance of non-flare to flare examples) are the default in the stella.FlareDataSet()
class. The only variables you must input is the directory to where you
are storing the light curves and the catalog.
Other variables that can be set are:
\(\textit{cadences}\): The number of cadences the CNN looks at at one time. Default = 200.
\(\textit{frac_balance}\): This fixes the class imbalances between the flare and no-flare classes. This is useful because we have a lot more no-flare cases and by rebalancing, we can train the CNN better. Default = 0.73.
\(\textit{training}\): The percentage of the data set that is set aside for training. The typical split is 80% for the training, 10% for the validation, and 10% for the test sets. Default = 0.80.
\(\textit{validation}\): The remaining percentage to be split between the validation and test sets after the training set has been assigned. Default = 0.90.
More information on these variables can be found in the API for stella.FlareDataSet().
If you downloaded the catalog through stella.DownloadSets()
you can initialize the FlareDataSet
class by calling:
ds = stella.FlareDataSet(downloadSet=download)
If you already have the catalog and light curves stored on your machine, you can call:
[21]:
ds = stella.FlareDataSet(fn_dir='/Users/arcticfox/Documents/flares/lc/unlabeled',
catalog='/Users/arcticfox/Documents/flares/lc/unlabeled/catalog_per_flare_final.csv')
Reading in training set files.
100%|██████████| 865/865 [00:01<00:00, 434.38it/s]
5389 positive classes (flare)
17684 negative classes (no flare)
30.0% class imbalance
If you did not use the DownloadSets
class, you can set the parameters fn_dir
and catalog
when initiating stella.FlareDataSet
.
The TQDM loading bar tracks which light curve files have been read in for creating the data set. \(\texttt{stella}\) will also print out the number of positive (flare) and negative (no flare) cases in the set as well as the class imbalance. Setting \(\textit{frac_balance} = 0.73\) results in an imbalance of 30%, which is recommended for training CNNs.
We can take a look at some of the flares and no flares in the training set data.
[22]:
ind_pc = np.where(ds.train_labels==1)[0] # Flares
ind_nc = np.where(ds.train_labels==0)[0] # No flares
fig, (ax1, ax2) = plt.subplots(ncols=2, figsize=(10,3),
sharex=True, sharey=True)
ax1.plot(ds.train_data[ind_pc[10]], 'r')
ax1.set_title('Flare')
ax1.set_xlabel('Cadences')
ax2.plot(ds.train_data[ind_nc[10]], 'k')
ax2.set_title('No Flare')
ax2.set_xlabel('Cadences');
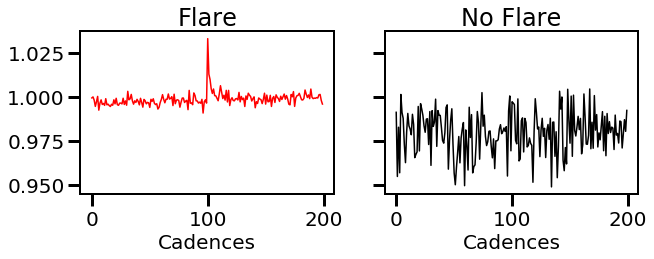
That definitely looks like a flare on the left and definitely doesn’t on the right!
1.2 Creating & Training a Model¶
Step 1. Specifiy a directory where you’d like your models to be saved to.
[23]:
OUT_DIR = '/Users/arcticfox/Desktop/results/'
Step 2. Initialize the class! Call \(\texttt{stella.ConvNN()}\) and pass in your directory and the \(\texttt{stella.DataSet}\) object. If you’re feeling adventerous, this is also the step where you can pass in a customized CNN architecture by passing in \(\textit{layers}\), and what \(\textit{optimizer}\), \(\textit{metrics}\), and \(\textit{loss}\) function you want to use. The default for each of these variables are described in the associated paper.
[24]:
cnn = stella.ConvNN(output_dir=OUT_DIR,
ds=ds)
To train your model, simply call \(\texttt{cnn.train_models()}\). By default, this will train a single model over 350 epochs and will pass in a batch size = 64 (which means the CNN will see 64 light curves at a time while training) and use an initial random seed = 2. It’s important to keep track of your random seeds so you can reproduce models later, if wanted. Calling this function will also predict on the validation set to give you an idea of how well your CNN is doing.
However, if you pass in a list of seeds, then this function will train len(seeds) many models over the same number of epochs. This is useful for \(\textit{ensembling}\), or running a bunch of models and averaging the predicted values over them.
The models you create will automatically be saved to your output directory in the following file format: ‘ensemble_s{0:04d}_i{1:04d}_b{2}.h5’.format(seed, epochs, frac_balance)
For this tutorial, we will train the CNN for 50 epochs, however we generally recommend training for \(\textbf{at least 300 epochs}\) or until signs of overfitting are seen in the metrics. More information on that below.
[29]:
cnn.train_models(seeds=2, epochs=200)
Model: "sequential"
_________________________________________________________________
Layer (type) Output Shape Param #
=================================================================
conv1d (Conv1D) (None, 200, 16) 128
_________________________________________________________________
max_pooling1d (MaxPooling1D) (None, 100, 16) 0
_________________________________________________________________
dropout (Dropout) (None, 100, 16) 0
_________________________________________________________________
conv1d_1 (Conv1D) (None, 100, 64) 3136
_________________________________________________________________
max_pooling1d_1 (MaxPooling1 (None, 50, 64) 0
_________________________________________________________________
dropout_1 (Dropout) (None, 50, 64) 0
_________________________________________________________________
flatten (Flatten) (None, 3200) 0
_________________________________________________________________
dense (Dense) (None, 32) 102432
_________________________________________________________________
dropout_2 (Dropout) (None, 32) 0
_________________________________________________________________
dense_1 (Dense) (None, 1) 33
=================================================================
Total params: 105,729
Trainable params: 105,729
Non-trainable params: 0
_________________________________________________________________
Train on 18458 samples, validate on 2307 samples
Epoch 1/200
18458/18458 [==============================] - 3s 174us/sample - loss: 0.5494 - accuracy: 0.7645 - precision: 0.2500 - recall: 2.3020e-04 - val_loss: 0.5289 - val_accuracy: 0.7707 - val_precision: 0.0000e+00 - val_recall: 0.0000e+00
Epoch 2/200
18458/18458 [==============================] - 2s 133us/sample - loss: 0.5324 - accuracy: 0.7647 - precision: 0.0000e+00 - recall: 0.0000e+00 - val_loss: 0.4919 - val_accuracy: 0.7707 - val_precision: 0.0000e+00 - val_recall: 0.0000e+00
Epoch 3/200
18458/18458 [==============================] - 2s 131us/sample - loss: 0.4737 - accuracy: 0.7863 - precision: 0.9761 - recall: 0.0942 - val_loss: 0.3863 - val_accuracy: 0.8466 - val_precision: 0.9944 - val_recall: 0.3327
Epoch 4/200
18458/18458 [==============================] - 3s 139us/sample - loss: 0.3604 - accuracy: 0.8620 - precision: 0.9653 - recall: 0.4291 - val_loss: 0.3166 - val_accuracy: 0.8643 - val_precision: 0.9865 - val_recall: 0.4140
Epoch 5/200
18458/18458 [==============================] - 3s 154us/sample - loss: 0.3120 - accuracy: 0.8820 - precision: 0.9577 - recall: 0.5216 - val_loss: 0.2419 - val_accuracy: 0.9016 - val_precision: 0.9809 - val_recall: 0.5822
Epoch 6/200
18458/18458 [==============================] - 3s 137us/sample - loss: 0.2938 - accuracy: 0.8948 - precision: 0.9475 - recall: 0.5854 - val_loss: 0.2647 - val_accuracy: 0.8882 - val_precision: 0.9892 - val_recall: 0.5180
Epoch 7/200
18458/18458 [==============================] - 3s 143us/sample - loss: 0.2628 - accuracy: 0.9055 - precision: 0.9514 - recall: 0.6308 - val_loss: 0.2178 - val_accuracy: 0.9137 - val_precision: 0.9797 - val_recall: 0.6371
Epoch 8/200
18458/18458 [==============================] - 2s 134us/sample - loss: 0.2715 - accuracy: 0.9031 - precision: 0.9422 - recall: 0.6268 - val_loss: 0.2429 - val_accuracy: 0.9068 - val_precision: 0.9816 - val_recall: 0.6049
Epoch 9/200
18458/18458 [==============================] - 3s 147us/sample - loss: 0.2567 - accuracy: 0.9083 - precision: 0.9311 - recall: 0.6593 - val_loss: 0.2139 - val_accuracy: 0.9272 - val_precision: 0.9640 - val_recall: 0.7089
Epoch 10/200
18458/18458 [==============================] - 2s 133us/sample - loss: 0.2447 - accuracy: 0.9140 - precision: 0.9230 - recall: 0.6924 - val_loss: 0.2234 - val_accuracy: 0.9272 - val_precision: 0.9593 - val_recall: 0.7127
Epoch 11/200
18458/18458 [==============================] - 2s 131us/sample - loss: 0.2286 - accuracy: 0.9192 - precision: 0.9242 - recall: 0.7155 - val_loss: 0.1910 - val_accuracy: 0.9332 - val_precision: 0.9518 - val_recall: 0.7467
Epoch 12/200
18458/18458 [==============================] - 2s 127us/sample - loss: 0.2241 - accuracy: 0.9227 - precision: 0.9244 - recall: 0.7316 - val_loss: 0.1881 - val_accuracy: 0.9276 - val_precision: 0.9525 - val_recall: 0.7202
Epoch 13/200
18458/18458 [==============================] - 2s 132us/sample - loss: 0.2025 - accuracy: 0.9306 - precision: 0.9327 - recall: 0.7599 - val_loss: 0.1686 - val_accuracy: 0.9371 - val_precision: 0.9444 - val_recall: 0.7713
Epoch 14/200
18458/18458 [==============================] - 2s 135us/sample - loss: 0.2141 - accuracy: 0.9288 - precision: 0.9314 - recall: 0.7530 - val_loss: 0.1662 - val_accuracy: 0.9371 - val_precision: 0.9528 - val_recall: 0.7637
Epoch 15/200
18458/18458 [==============================] - 3s 142us/sample - loss: 0.2016 - accuracy: 0.9320 - precision: 0.9282 - recall: 0.7705 - val_loss: 0.2023 - val_accuracy: 0.9224 - val_precision: 0.9730 - val_recall: 0.6805
Epoch 16/200
18458/18458 [==============================] - 3s 138us/sample - loss: 0.2043 - accuracy: 0.9303 - precision: 0.9245 - recall: 0.7666 - val_loss: 0.1942 - val_accuracy: 0.9306 - val_precision: 0.9792 - val_recall: 0.7127
Epoch 17/200
18458/18458 [==============================] - 2s 132us/sample - loss: 0.1916 - accuracy: 0.9362 - precision: 0.9366 - recall: 0.7820 - val_loss: 0.1506 - val_accuracy: 0.9458 - val_precision: 0.9633 - val_recall: 0.7940
Epoch 18/200
18458/18458 [==============================] - 2s 135us/sample - loss: 0.1894 - accuracy: 0.9370 - precision: 0.9364 - recall: 0.7857 - val_loss: 0.1658 - val_accuracy: 0.9363 - val_precision: 0.9848 - val_recall: 0.7335
Epoch 19/200
18458/18458 [==============================] - 3s 144us/sample - loss: 0.1748 - accuracy: 0.9426 - precision: 0.9460 - recall: 0.8020 - val_loss: 0.1541 - val_accuracy: 0.9450 - val_precision: 0.9855 - val_recall: 0.7713
Epoch 20/200
18458/18458 [==============================] - 3s 143us/sample - loss: 0.1772 - accuracy: 0.9430 - precision: 0.9487 - recall: 0.8011 - val_loss: 0.1432 - val_accuracy: 0.9484 - val_precision: 0.9724 - val_recall: 0.7977
Epoch 21/200
18458/18458 [==============================] - 2s 134us/sample - loss: 0.1881 - accuracy: 0.9385 - precision: 0.9378 - recall: 0.7912 - val_loss: 0.1620 - val_accuracy: 0.9402 - val_precision: 0.9780 - val_recall: 0.7561
Epoch 22/200
18458/18458 [==============================] - 3s 136us/sample - loss: 0.1757 - accuracy: 0.9428 - precision: 0.9489 - recall: 0.8002 - val_loss: 0.1311 - val_accuracy: 0.9567 - val_precision: 0.9673 - val_recall: 0.8393
Epoch 23/200
18458/18458 [==============================] - 3s 142us/sample - loss: 0.1633 - accuracy: 0.9491 - precision: 0.9568 - recall: 0.8207 - val_loss: 0.1282 - val_accuracy: 0.9575 - val_precision: 0.9615 - val_recall: 0.8488
Epoch 24/200
18458/18458 [==============================] - 2s 129us/sample - loss: 0.1653 - accuracy: 0.9467 - precision: 0.9546 - recall: 0.8124 - val_loss: 0.1277 - val_accuracy: 0.9645 - val_precision: 0.9786 - val_recall: 0.8639
Epoch 25/200
18458/18458 [==============================] - 3s 138us/sample - loss: 0.1575 - accuracy: 0.9516 - precision: 0.9600 - recall: 0.8290 - val_loss: 0.1345 - val_accuracy: 0.9714 - val_precision: 0.9715 - val_recall: 0.9017
Epoch 26/200
18458/18458 [==============================] - 3s 136us/sample - loss: 0.1517 - accuracy: 0.9518 - precision: 0.9569 - recall: 0.8326 - val_loss: 0.1194 - val_accuracy: 0.9718 - val_precision: 0.9696 - val_recall: 0.9055
Epoch 27/200
18458/18458 [==============================] - 2s 134us/sample - loss: 0.1546 - accuracy: 0.9519 - precision: 0.9567 - recall: 0.8336 - val_loss: 0.1546 - val_accuracy: 0.9714 - val_precision: 0.9530 - val_recall: 0.9206
Epoch 28/200
18458/18458 [==============================] - 3s 136us/sample - loss: 0.1399 - accuracy: 0.9561 - precision: 0.9614 - recall: 0.8476 - val_loss: 0.1711 - val_accuracy: 0.9710 - val_precision: 0.9262 - val_recall: 0.9490
Epoch 29/200
18458/18458 [==============================] - 2s 132us/sample - loss: 0.1476 - accuracy: 0.9546 - precision: 0.9599 - recall: 0.8423 - val_loss: 0.1175 - val_accuracy: 0.9632 - val_precision: 0.9723 - val_recall: 0.8639
Epoch 30/200
18458/18458 [==============================] - 2s 133us/sample - loss: 0.1420 - accuracy: 0.9562 - precision: 0.9585 - recall: 0.8506 - val_loss: 0.1119 - val_accuracy: 0.9697 - val_precision: 0.9713 - val_recall: 0.8941
Epoch 31/200
18458/18458 [==============================] - 2s 133us/sample - loss: 0.1488 - accuracy: 0.9539 - precision: 0.9561 - recall: 0.8430 - val_loss: 0.1121 - val_accuracy: 0.9736 - val_precision: 0.9680 - val_recall: 0.9149
Epoch 32/200
18458/18458 [==============================] - 2s 134us/sample - loss: 0.1413 - accuracy: 0.9567 - precision: 0.9624 - recall: 0.8492 - val_loss: 0.1030 - val_accuracy: 0.9701 - val_precision: 0.9733 - val_recall: 0.8941
Epoch 33/200
18458/18458 [==============================] - 2s 134us/sample - loss: 0.1398 - accuracy: 0.9547 - precision: 0.9580 - recall: 0.8444 - val_loss: 0.1127 - val_accuracy: 0.9775 - val_precision: 0.9649 - val_recall: 0.9357
Epoch 34/200
18458/18458 [==============================] - 3s 150us/sample - loss: 0.1326 - accuracy: 0.9582 - precision: 0.9625 - recall: 0.8559 - val_loss: 0.1092 - val_accuracy: 0.9775 - val_precision: 0.9704 - val_recall: 0.9301
Epoch 35/200
18458/18458 [==============================] - 3s 186us/sample - loss: 0.1385 - accuracy: 0.9577 - precision: 0.9643 - recall: 0.8517 - val_loss: 0.1370 - val_accuracy: 0.9740 - val_precision: 0.9383 - val_recall: 0.9490
Epoch 36/200
18458/18458 [==============================] - 4s 207us/sample - loss: 0.1301 - accuracy: 0.9604 - precision: 0.9672 - recall: 0.8610 - val_loss: 0.1323 - val_accuracy: 0.9567 - val_precision: 0.9633 - val_recall: 0.8431
Epoch 37/200
18458/18458 [==============================] - 4s 192us/sample - loss: 0.1275 - accuracy: 0.9605 - precision: 0.9626 - recall: 0.8658 - val_loss: 0.1484 - val_accuracy: 0.9749 - val_precision: 0.9305 - val_recall: 0.9622
Epoch 38/200
18458/18458 [==============================] - 3s 168us/sample - loss: 0.1423 - accuracy: 0.9550 - precision: 0.9549 - recall: 0.8490 - val_loss: 0.1096 - val_accuracy: 0.9684 - val_precision: 0.9653 - val_recall: 0.8941
Epoch 39/200
18458/18458 [==============================] - 3s 146us/sample - loss: 0.1412 - accuracy: 0.9543 - precision: 0.9548 - recall: 0.8460 - val_loss: 0.1397 - val_accuracy: 0.9701 - val_precision: 0.9212 - val_recall: 0.9509
Epoch 40/200
18458/18458 [==============================] - 3s 138us/sample - loss: 0.1285 - accuracy: 0.9598 - precision: 0.9649 - recall: 0.8605 - val_loss: 0.1038 - val_accuracy: 0.9679 - val_precision: 0.9577 - val_recall: 0.8998
Epoch 41/200
18458/18458 [==============================] - 3s 144us/sample - loss: 0.1324 - accuracy: 0.9584 - precision: 0.9625 - recall: 0.8568 - val_loss: 0.1238 - val_accuracy: 0.9757 - val_precision: 0.9355 - val_recall: 0.9603
Epoch 42/200
18458/18458 [==============================] - 3s 142us/sample - loss: 0.1273 - accuracy: 0.9613 - precision: 0.9635 - recall: 0.8686 - val_loss: 0.2219 - val_accuracy: 0.9376 - val_precision: 0.7966 - val_recall: 0.9773
Epoch 43/200
18458/18458 [==============================] - 3s 145us/sample - loss: 0.1220 - accuracy: 0.9625 - precision: 0.9670 - recall: 0.8702 - val_loss: 0.0966 - val_accuracy: 0.9701 - val_precision: 0.9832 - val_recall: 0.8847
Epoch 44/200
18458/18458 [==============================] - 3s 152us/sample - loss: 0.1265 - accuracy: 0.9610 - precision: 0.9658 - recall: 0.8651 - val_loss: 0.0997 - val_accuracy: 0.9697 - val_precision: 0.9752 - val_recall: 0.8904
Epoch 45/200
18458/18458 [==============================] - 3s 143us/sample - loss: 0.1268 - accuracy: 0.9615 - precision: 0.9633 - recall: 0.8695 - val_loss: 0.0941 - val_accuracy: 0.9710 - val_precision: 0.9676 - val_recall: 0.9036
Epoch 46/200
18458/18458 [==============================] - 3s 149us/sample - loss: 0.1285 - accuracy: 0.9601 - precision: 0.9635 - recall: 0.8633 - val_loss: 0.1479 - val_accuracy: 0.9684 - val_precision: 0.9028 - val_recall: 0.9660
Epoch 47/200
18458/18458 [==============================] - 3s 143us/sample - loss: 0.1328 - accuracy: 0.9585 - precision: 0.9571 - recall: 0.8623 - val_loss: 0.1346 - val_accuracy: 0.9658 - val_precision: 0.9151 - val_recall: 0.9376
Epoch 48/200
18458/18458 [==============================] - 3s 137us/sample - loss: 0.1177 - accuracy: 0.9627 - precision: 0.9668 - recall: 0.8713 - val_loss: 0.1043 - val_accuracy: 0.9770 - val_precision: 0.9440 - val_recall: 0.9565
Epoch 49/200
18458/18458 [==============================] - 3s 144us/sample - loss: 0.1247 - accuracy: 0.9613 - precision: 0.9649 - recall: 0.8669 - val_loss: 0.0888 - val_accuracy: 0.9705 - val_precision: 0.9792 - val_recall: 0.8904
Epoch 50/200
18458/18458 [==============================] - 3s 159us/sample - loss: 0.1171 - accuracy: 0.9642 - precision: 0.9668 - recall: 0.8780 - val_loss: 0.0991 - val_accuracy: 0.9701 - val_precision: 0.9812 - val_recall: 0.8866
Epoch 51/200
18458/18458 [==============================] - 3s 169us/sample - loss: 0.1190 - accuracy: 0.9638 - precision: 0.9674 - recall: 0.8755 - val_loss: 0.1182 - val_accuracy: 0.9723 - val_precision: 0.9204 - val_recall: 0.9622
Epoch 52/200
18458/18458 [==============================] - 3s 161us/sample - loss: 0.1179 - accuracy: 0.9628 - precision: 0.9635 - recall: 0.8752 - val_loss: 0.1217 - val_accuracy: 0.9627 - val_precision: 0.9743 - val_recall: 0.8601
Epoch 53/200
18458/18458 [==============================] - 3s 150us/sample - loss: 0.1166 - accuracy: 0.9632 - precision: 0.9671 - recall: 0.8734 - val_loss: 0.1692 - val_accuracy: 0.9649 - val_precision: 0.8836 - val_recall: 0.9754
Epoch 54/200
18458/18458 [==============================] - 3s 138us/sample - loss: 0.1164 - accuracy: 0.9627 - precision: 0.9661 - recall: 0.8720 - val_loss: 0.0974 - val_accuracy: 0.9783 - val_precision: 0.9460 - val_recall: 0.9603
Epoch 55/200
18458/18458 [==============================] - 2s 135us/sample - loss: 0.1215 - accuracy: 0.9627 - precision: 0.9659 - recall: 0.8725 - val_loss: 0.1093 - val_accuracy: 0.9766 - val_precision: 0.9406 - val_recall: 0.9584
Epoch 56/200
18458/18458 [==============================] - 3s 138us/sample - loss: 0.1217 - accuracy: 0.9619 - precision: 0.9636 - recall: 0.8709 - val_loss: 0.0962 - val_accuracy: 0.9783 - val_precision: 0.9460 - val_recall: 0.9603
Epoch 57/200
18458/18458 [==============================] - 3s 144us/sample - loss: 0.1147 - accuracy: 0.9635 - precision: 0.9672 - recall: 0.8748 - val_loss: 0.1161 - val_accuracy: 0.9744 - val_precision: 0.9288 - val_recall: 0.9622
Epoch 58/200
18458/18458 [==============================] - 3s 141us/sample - loss: 0.1266 - accuracy: 0.9596 - precision: 0.9610 - recall: 0.8633 - val_loss: 0.1003 - val_accuracy: 0.9697 - val_precision: 0.9674 - val_recall: 0.8979
Epoch 59/200
18458/18458 [==============================] - 3s 145us/sample - loss: 0.1082 - accuracy: 0.9647 - precision: 0.9641 - recall: 0.8831 - val_loss: 0.0879 - val_accuracy: 0.9710 - val_precision: 0.9833 - val_recall: 0.8885
Epoch 60/200
18458/18458 [==============================] - 3s 142us/sample - loss: 0.1127 - accuracy: 0.9633 - precision: 0.9636 - recall: 0.8773 - val_loss: 0.0904 - val_accuracy: 0.9727 - val_precision: 0.9854 - val_recall: 0.8941
Epoch 61/200
18458/18458 [==============================] - 3s 143us/sample - loss: 0.1044 - accuracy: 0.9679 - precision: 0.9688 - recall: 0.8923 - val_loss: 0.1206 - val_accuracy: 0.9688 - val_precision: 0.9044 - val_recall: 0.9660
Epoch 62/200
18458/18458 [==============================] - 3s 143us/sample - loss: 0.1095 - accuracy: 0.9641 - precision: 0.9619 - recall: 0.8824 - val_loss: 0.1610 - val_accuracy: 0.9545 - val_precision: 0.8522 - val_recall: 0.9698
Epoch 63/200
18458/18458 [==============================] - 3s 140us/sample - loss: 0.0996 - accuracy: 0.9681 - precision: 0.9686 - recall: 0.8936 - val_loss: 0.0970 - val_accuracy: 0.9775 - val_precision: 0.9425 - val_recall: 0.9603
Epoch 64/200
18458/18458 [==============================] - 2s 135us/sample - loss: 0.1073 - accuracy: 0.9670 - precision: 0.9670 - recall: 0.8900 - val_loss: 0.0922 - val_accuracy: 0.9744 - val_precision: 0.9368 - val_recall: 0.9527
Epoch 65/200
18458/18458 [==============================] - 2s 133us/sample - loss: 0.1280 - accuracy: 0.9597 - precision: 0.9658 - recall: 0.8591 - val_loss: 0.1154 - val_accuracy: 0.9688 - val_precision: 0.9117 - val_recall: 0.9565
Epoch 66/200
18458/18458 [==============================] - 2s 133us/sample - loss: 0.1054 - accuracy: 0.9672 - precision: 0.9722 - recall: 0.8860 - val_loss: 0.0749 - val_accuracy: 0.9766 - val_precision: 0.9612 - val_recall: 0.9357
Epoch 67/200
18458/18458 [==============================] - 2s 132us/sample - loss: 0.1024 - accuracy: 0.9691 - precision: 0.9682 - recall: 0.8983 - val_loss: 0.0882 - val_accuracy: 0.9723 - val_precision: 0.9412 - val_recall: 0.9376
Epoch 68/200
18458/18458 [==============================] - 2s 133us/sample - loss: 0.1017 - accuracy: 0.9684 - precision: 0.9709 - recall: 0.8923 - val_loss: 0.0765 - val_accuracy: 0.9792 - val_precision: 0.9529 - val_recall: 0.9565
Epoch 69/200
18458/18458 [==============================] - 2s 133us/sample - loss: 0.1056 - accuracy: 0.9665 - precision: 0.9662 - recall: 0.8886 - val_loss: 0.1364 - val_accuracy: 0.9645 - val_precision: 0.8847 - val_recall: 0.9716
Epoch 70/200
18458/18458 [==============================] - 2s 133us/sample - loss: 0.1099 - accuracy: 0.9668 - precision: 0.9670 - recall: 0.8893 - val_loss: 0.1352 - val_accuracy: 0.9619 - val_precision: 0.8769 - val_recall: 0.9698
Epoch 71/200
18458/18458 [==============================] - 3s 136us/sample - loss: 0.1013 - accuracy: 0.9669 - precision: 0.9670 - recall: 0.8897 - val_loss: 0.0828 - val_accuracy: 0.9796 - val_precision: 0.9513 - val_recall: 0.9603
Epoch 72/200
18458/18458 [==============================] - 3s 136us/sample - loss: 0.1045 - accuracy: 0.9680 - precision: 0.9695 - recall: 0.8923 - val_loss: 0.0694 - val_accuracy: 0.9766 - val_precision: 0.9837 - val_recall: 0.9130
Epoch 73/200
18458/18458 [==============================] - 2s 135us/sample - loss: 0.1014 - accuracy: 0.9668 - precision: 0.9674 - recall: 0.8888 - val_loss: 0.1153 - val_accuracy: 0.9671 - val_precision: 0.8953 - val_recall: 0.9698
Epoch 74/200
18458/18458 [==============================] - 3s 136us/sample - loss: 0.1091 - accuracy: 0.9641 - precision: 0.9663 - recall: 0.8782 - val_loss: 0.0874 - val_accuracy: 0.9753 - val_precision: 0.9664 - val_recall: 0.9244
Epoch 75/200
18458/18458 [==============================] - 3s 136us/sample - loss: 0.0947 - accuracy: 0.9706 - precision: 0.9698 - recall: 0.9031 - val_loss: 0.1204 - val_accuracy: 0.9593 - val_precision: 0.9801 - val_recall: 0.8393
Epoch 76/200
18458/18458 [==============================] - 2s 134us/sample - loss: 0.1010 - accuracy: 0.9691 - precision: 0.9720 - recall: 0.8946 - val_loss: 0.0929 - val_accuracy: 0.9783 - val_precision: 0.9362 - val_recall: 0.9716
Epoch 77/200
18458/18458 [==============================] - 2s 131us/sample - loss: 0.0931 - accuracy: 0.9709 - precision: 0.9685 - recall: 0.9058 - val_loss: 0.0737 - val_accuracy: 0.9770 - val_precision: 0.9837 - val_recall: 0.9149
Epoch 78/200
18458/18458 [==============================] - 2s 131us/sample - loss: 0.0983 - accuracy: 0.9686 - precision: 0.9698 - recall: 0.8946 - val_loss: 0.0979 - val_accuracy: 0.9757 - val_precision: 0.9261 - val_recall: 0.9716
Epoch 79/200
18458/18458 [==============================] - 2s 131us/sample - loss: 0.0980 - accuracy: 0.9687 - precision: 0.9712 - recall: 0.8936 - val_loss: 0.0732 - val_accuracy: 0.9753 - val_precision: 0.9609 - val_recall: 0.9301
Epoch 80/200
18458/18458 [==============================] - 2s 131us/sample - loss: 0.0974 - accuracy: 0.9698 - precision: 0.9704 - recall: 0.8989 - val_loss: 0.3404 - val_accuracy: 0.8331 - val_precision: 0.5793 - val_recall: 0.9943
Epoch 81/200
18458/18458 [==============================] - 2s 133us/sample - loss: 0.0957 - accuracy: 0.9685 - precision: 0.9649 - recall: 0.8989 - val_loss: 0.0779 - val_accuracy: 0.9801 - val_precision: 0.9514 - val_recall: 0.9622
Epoch 82/200
18458/18458 [==============================] - 2s 135us/sample - loss: 0.0977 - accuracy: 0.9691 - precision: 0.9689 - recall: 0.8976 - val_loss: 0.1189 - val_accuracy: 0.9636 - val_precision: 0.8843 - val_recall: 0.9679
Epoch 83/200
18458/18458 [==============================] - 2s 135us/sample - loss: 0.1032 - accuracy: 0.9686 - precision: 0.9724 - recall: 0.8918 - val_loss: 0.1481 - val_accuracy: 0.9523 - val_precision: 0.8463 - val_recall: 0.9679
Epoch 84/200
18458/18458 [==============================] - 2s 135us/sample - loss: 0.0898 - accuracy: 0.9713 - precision: 0.9706 - recall: 0.9056 - val_loss: 0.1526 - val_accuracy: 0.9549 - val_precision: 0.8489 - val_recall: 0.9773
Epoch 85/200
18458/18458 [==============================] - 3s 135us/sample - loss: 0.0930 - accuracy: 0.9711 - precision: 0.9694 - recall: 0.9056 - val_loss: 0.0901 - val_accuracy: 0.9783 - val_precision: 0.9378 - val_recall: 0.9698
Epoch 86/200
18458/18458 [==============================] - 3s 138us/sample - loss: 0.0908 - accuracy: 0.9713 - precision: 0.9695 - recall: 0.9065 - val_loss: 0.0664 - val_accuracy: 0.9792 - val_precision: 0.9598 - val_recall: 0.9490
Epoch 87/200
18458/18458 [==============================] - 3s 136us/sample - loss: 0.0961 - accuracy: 0.9710 - precision: 0.9708 - recall: 0.9038 - val_loss: 0.1742 - val_accuracy: 0.9480 - val_precision: 0.8231 - val_recall: 0.9849
Epoch 88/200
18458/18458 [==============================] - 2s 133us/sample - loss: 0.0930 - accuracy: 0.9714 - precision: 0.9723 - recall: 0.9045 - val_loss: 0.0733 - val_accuracy: 0.9766 - val_precision: 0.9507 - val_recall: 0.9471
Epoch 89/200
18458/18458 [==============================] - 2s 133us/sample - loss: 0.0891 - accuracy: 0.9719 - precision: 0.9705 - recall: 0.9084 - val_loss: 0.0863 - val_accuracy: 0.9710 - val_precision: 0.9894 - val_recall: 0.8828
Epoch 90/200
18458/18458 [==============================] - 2s 133us/sample - loss: 0.0916 - accuracy: 0.9716 - precision: 0.9714 - recall: 0.9061 - val_loss: 0.0992 - val_accuracy: 0.9766 - val_precision: 0.9295 - val_recall: 0.9716
Epoch 91/200
18458/18458 [==============================] - 2s 133us/sample - loss: 0.0935 - accuracy: 0.9716 - precision: 0.9704 - recall: 0.9068 - val_loss: 0.0771 - val_accuracy: 0.9792 - val_precision: 0.9512 - val_recall: 0.9584
Epoch 92/200
18458/18458 [==============================] - 2s 133us/sample - loss: 0.0949 - accuracy: 0.9701 - precision: 0.9705 - recall: 0.9003 - val_loss: 0.1643 - val_accuracy: 0.9480 - val_precision: 0.8251 - val_recall: 0.9811
Epoch 93/200
18458/18458 [==============================] - 3s 148us/sample - loss: 0.0939 - accuracy: 0.9699 - precision: 0.9707 - recall: 0.8994 - val_loss: 0.1503 - val_accuracy: 0.9536 - val_precision: 0.8436 - val_recall: 0.9792
Epoch 94/200
18458/18458 [==============================] - 3s 174us/sample - loss: 0.0853 - accuracy: 0.9726 - precision: 0.9722 - recall: 0.9095 - val_loss: 0.0682 - val_accuracy: 0.9809 - val_precision: 0.9550 - val_recall: 0.9622
Epoch 95/200
18458/18458 [==============================] - 3s 172us/sample - loss: 0.0918 - accuracy: 0.9735 - precision: 0.9742 - recall: 0.9114 - val_loss: 0.1143 - val_accuracy: 0.9675 - val_precision: 0.8914 - val_recall: 0.9773
Epoch 96/200
18458/18458 [==============================] - 3s 166us/sample - loss: 0.0991 - accuracy: 0.9674 - precision: 0.9636 - recall: 0.8953 - val_loss: 0.1964 - val_accuracy: 0.9380 - val_precision: 0.7915 - val_recall: 0.9905
Epoch 97/200
18458/18458 [==============================] - 3s 160us/sample - loss: 0.0984 - accuracy: 0.9678 - precision: 0.9659 - recall: 0.8946 - val_loss: 0.0652 - val_accuracy: 0.9814 - val_precision: 0.9602 - val_recall: 0.9584
Epoch 98/200
18458/18458 [==============================] - 3s 139us/sample - loss: 0.0891 - accuracy: 0.9730 - precision: 0.9722 - recall: 0.9111 - val_loss: 0.0734 - val_accuracy: 0.9809 - val_precision: 0.9482 - val_recall: 0.9698
Epoch 99/200
18458/18458 [==============================] - 2s 135us/sample - loss: 0.0890 - accuracy: 0.9712 - precision: 0.9681 - recall: 0.9077 - val_loss: 0.1848 - val_accuracy: 0.9410 - val_precision: 0.8028 - val_recall: 0.9849
Epoch 100/200
18458/18458 [==============================] - 2s 134us/sample - loss: 0.0870 - accuracy: 0.9724 - precision: 0.9717 - recall: 0.9091 - val_loss: 0.1258 - val_accuracy: 0.9619 - val_precision: 0.8718 - val_recall: 0.9773
Epoch 101/200
18458/18458 [==============================] - 2s 133us/sample - loss: 0.0971 - accuracy: 0.9697 - precision: 0.9699 - recall: 0.8989 - val_loss: 0.0624 - val_accuracy: 0.9788 - val_precision: 0.9800 - val_recall: 0.9263
Epoch 102/200
18458/18458 [==============================] - 2s 132us/sample - loss: 0.0995 - accuracy: 0.9691 - precision: 0.9696 - recall: 0.8966 - val_loss: 0.0798 - val_accuracy: 0.9736 - val_precision: 0.9916 - val_recall: 0.8922
Epoch 103/200
18458/18458 [==============================] - 3s 143us/sample - loss: 0.0925 - accuracy: 0.9718 - precision: 0.9733 - recall: 0.9049 - val_loss: 0.0652 - val_accuracy: 0.9818 - val_precision: 0.9586 - val_recall: 0.9622
Epoch 104/200
18458/18458 [==============================] - 3s 141us/sample - loss: 0.0911 - accuracy: 0.9705 - precision: 0.9701 - recall: 0.9026 - val_loss: 0.1040 - val_accuracy: 0.9697 - val_precision: 0.8991 - val_recall: 0.9773
Epoch 105/200
18458/18458 [==============================] - 3s 141us/sample - loss: 0.0922 - accuracy: 0.9706 - precision: 0.9694 - recall: 0.9035 - val_loss: 0.1581 - val_accuracy: 0.9519 - val_precision: 0.8382 - val_recall: 0.9792
Epoch 106/200
18458/18458 [==============================] - 3s 139us/sample - loss: 0.1069 - accuracy: 0.9667 - precision: 0.9635 - recall: 0.8925 - val_loss: 0.1421 - val_accuracy: 0.9567 - val_precision: 0.8557 - val_recall: 0.9754
Epoch 107/200
18458/18458 [==============================] - 3s 141us/sample - loss: 0.0930 - accuracy: 0.9710 - precision: 0.9720 - recall: 0.9029 - val_loss: 0.1410 - val_accuracy: 0.9562 - val_precision: 0.8543 - val_recall: 0.9754
Epoch 108/200
18458/18458 [==============================] - 3s 139us/sample - loss: 0.0886 - accuracy: 0.9722 - precision: 0.9735 - recall: 0.9065 - val_loss: 0.1257 - val_accuracy: 0.9627 - val_precision: 0.8761 - val_recall: 0.9754
Epoch 109/200
18458/18458 [==============================] - 3s 136us/sample - loss: 0.0888 - accuracy: 0.9717 - precision: 0.9712 - recall: 0.9068 - val_loss: 0.0702 - val_accuracy: 0.9818 - val_precision: 0.9534 - val_recall: 0.9679
Epoch 110/200
18458/18458 [==============================] - 3s 138us/sample - loss: 0.0936 - accuracy: 0.9711 - precision: 0.9722 - recall: 0.9029 - val_loss: 0.0883 - val_accuracy: 0.9749 - val_precision: 0.9213 - val_recall: 0.9735
Epoch 111/200
18458/18458 [==============================] - 3s 138us/sample - loss: 0.0896 - accuracy: 0.9719 - precision: 0.9712 - recall: 0.9077 - val_loss: 0.0853 - val_accuracy: 0.9775 - val_precision: 0.9328 - val_recall: 0.9716
Epoch 112/200
18458/18458 [==============================] - 2s 135us/sample - loss: 0.0909 - accuracy: 0.9724 - precision: 0.9710 - recall: 0.9098 - val_loss: 0.1586 - val_accuracy: 0.9523 - val_precision: 0.8429 - val_recall: 0.9735
Epoch 113/200
18458/18458 [==============================] - 2s 135us/sample - loss: 0.0875 - accuracy: 0.9717 - precision: 0.9719 - recall: 0.9061 - val_loss: 0.0689 - val_accuracy: 0.9809 - val_precision: 0.9602 - val_recall: 0.9565
Epoch 114/200
18458/18458 [==============================] - 3s 153us/sample - loss: 0.0836 - accuracy: 0.9737 - precision: 0.9758 - recall: 0.9107 - val_loss: 0.0657 - val_accuracy: 0.9818 - val_precision: 0.9518 - val_recall: 0.9698
Epoch 115/200
18458/18458 [==============================] - 3s 142us/sample - loss: 0.0921 - accuracy: 0.9711 - precision: 0.9708 - recall: 0.9042 - val_loss: 0.0702 - val_accuracy: 0.9805 - val_precision: 0.9498 - val_recall: 0.9660
Epoch 116/200
18458/18458 [==============================] - 3s 140us/sample - loss: 0.0974 - accuracy: 0.9690 - precision: 0.9671 - recall: 0.8987 - val_loss: 0.1202 - val_accuracy: 0.9649 - val_precision: 0.8875 - val_recall: 0.9698
Epoch 117/200
18458/18458 [==============================] - 3s 136us/sample - loss: 0.0850 - accuracy: 0.9722 - precision: 0.9717 - recall: 0.9084 - val_loss: 0.1045 - val_accuracy: 0.9714 - val_precision: 0.9069 - val_recall: 0.9754
Epoch 118/200
18458/18458 [==============================] - 3s 162us/sample - loss: 0.0857 - accuracy: 0.9741 - precision: 0.9733 - recall: 0.9151 - val_loss: 0.0758 - val_accuracy: 0.9809 - val_precision: 0.9499 - val_recall: 0.9679
Epoch 119/200
18458/18458 [==============================] - 3s 156us/sample - loss: 0.0863 - accuracy: 0.9729 - precision: 0.9729 - recall: 0.9100 - val_loss: 0.2024 - val_accuracy: 0.9315 - val_precision: 0.7732 - val_recall: 0.9924
Epoch 120/200
18458/18458 [==============================] - 3s 155us/sample - loss: 0.1031 - accuracy: 0.9690 - precision: 0.9694 - recall: 0.8966 - val_loss: 0.0815 - val_accuracy: 0.9705 - val_precision: 0.9458 - val_recall: 0.9244
Epoch 121/200
18458/18458 [==============================] - 3s 155us/sample - loss: 0.0880 - accuracy: 0.9724 - precision: 0.9712 - recall: 0.9098 - val_loss: 0.1191 - val_accuracy: 0.9662 - val_precision: 0.9005 - val_recall: 0.9584
Epoch 122/200
18458/18458 [==============================] - 3s 145us/sample - loss: 0.0942 - accuracy: 0.9706 - precision: 0.9684 - recall: 0.9045 - val_loss: 0.1026 - val_accuracy: 0.9697 - val_precision: 0.9005 - val_recall: 0.9754
Epoch 123/200
18458/18458 [==============================] - 3s 137us/sample - loss: 0.0876 - accuracy: 0.9722 - precision: 0.9735 - recall: 0.9065 - val_loss: 0.0634 - val_accuracy: 0.9814 - val_precision: 0.9620 - val_recall: 0.9565
Epoch 124/200
18458/18458 [==============================] - 3s 145us/sample - loss: 0.0854 - accuracy: 0.9725 - precision: 0.9715 - recall: 0.9098 - val_loss: 0.0870 - val_accuracy: 0.9736 - val_precision: 0.9179 - val_recall: 0.9716
Epoch 125/200
18458/18458 [==============================] - 3s 147us/sample - loss: 0.0901 - accuracy: 0.9721 - precision: 0.9717 - recall: 0.9079 - val_loss: 0.1402 - val_accuracy: 0.9541 - val_precision: 0.8484 - val_recall: 0.9735
Epoch 126/200
18458/18458 [==============================] - 3s 137us/sample - loss: 0.0903 - accuracy: 0.9718 - precision: 0.9712 - recall: 0.9072 - val_loss: 0.1050 - val_accuracy: 0.9666 - val_precision: 0.8979 - val_recall: 0.9641
Epoch 127/200
18458/18458 [==============================] - 2s 133us/sample - loss: 0.0899 - accuracy: 0.9718 - precision: 0.9721 - recall: 0.9063 - val_loss: 0.0627 - val_accuracy: 0.9775 - val_precision: 0.9818 - val_recall: 0.9187
Epoch 128/200
18458/18458 [==============================] - 3s 148us/sample - loss: 0.0909 - accuracy: 0.9714 - precision: 0.9709 - recall: 0.9056 - val_loss: 0.1331 - val_accuracy: 0.9619 - val_precision: 0.8693 - val_recall: 0.9811
Epoch 129/200
18458/18458 [==============================] - 3s 140us/sample - loss: 0.0987 - accuracy: 0.9680 - precision: 0.9681 - recall: 0.8936 - val_loss: 0.1068 - val_accuracy: 0.9666 - val_precision: 0.8897 - val_recall: 0.9754
Epoch 130/200
18458/18458 [==============================] - 3s 140us/sample - loss: 0.0808 - accuracy: 0.9746 - precision: 0.9745 - recall: 0.9160 - val_loss: 0.0795 - val_accuracy: 0.9753 - val_precision: 0.9260 - val_recall: 0.9698
Epoch 131/200
18458/18458 [==============================] - 3s 140us/sample - loss: 0.0899 - accuracy: 0.9722 - precision: 0.9698 - recall: 0.9100 - val_loss: 0.1077 - val_accuracy: 0.9623 - val_precision: 0.8932 - val_recall: 0.9490
Epoch 132/200
18458/18458 [==============================] - 2s 135us/sample - loss: 0.0853 - accuracy: 0.9739 - precision: 0.9731 - recall: 0.9146 - val_loss: 0.1118 - val_accuracy: 0.9627 - val_precision: 0.8799 - val_recall: 0.9698
Epoch 133/200
18458/18458 [==============================] - 2s 131us/sample - loss: 0.0965 - accuracy: 0.9697 - precision: 0.9690 - recall: 0.8999 - val_loss: 0.1128 - val_accuracy: 0.9575 - val_precision: 0.8954 - val_recall: 0.9225
Epoch 134/200
18458/18458 [==============================] - 2s 130us/sample - loss: 0.0885 - accuracy: 0.9717 - precision: 0.9682 - recall: 0.9098 - val_loss: 0.0859 - val_accuracy: 0.9701 - val_precision: 0.9064 - val_recall: 0.9698
Epoch 135/200
18458/18458 [==============================] - 2s 129us/sample - loss: 0.0915 - accuracy: 0.9706 - precision: 0.9682 - recall: 0.9049 - val_loss: 0.0814 - val_accuracy: 0.9749 - val_precision: 0.9274 - val_recall: 0.9660
Epoch 136/200
18458/18458 [==============================] - 2s 129us/sample - loss: 0.0927 - accuracy: 0.9712 - precision: 0.9699 - recall: 0.9058 - val_loss: 0.1020 - val_accuracy: 0.9688 - val_precision: 0.8988 - val_recall: 0.9735
Epoch 137/200
18458/18458 [==============================] - 2s 131us/sample - loss: 0.0865 - accuracy: 0.9732 - precision: 0.9725 - recall: 0.9121 - val_loss: 0.0615 - val_accuracy: 0.9822 - val_precision: 0.9552 - val_recall: 0.9679
Epoch 138/200
18458/18458 [==============================] - 2s 133us/sample - loss: 0.0812 - accuracy: 0.9752 - precision: 0.9741 - recall: 0.9192 - val_loss: 0.0655 - val_accuracy: 0.9801 - val_precision: 0.9497 - val_recall: 0.9641
Epoch 139/200
18458/18458 [==============================] - 3s 137us/sample - loss: 0.0857 - accuracy: 0.9737 - precision: 0.9749 - recall: 0.9116 - val_loss: 0.1483 - val_accuracy: 0.9536 - val_precision: 0.8403 - val_recall: 0.9849
Epoch 140/200
18458/18458 [==============================] - 3s 138us/sample - loss: 0.1002 - accuracy: 0.9686 - precision: 0.9682 - recall: 0.8959 - val_loss: 0.1285 - val_accuracy: 0.9610 - val_precision: 0.8702 - val_recall: 0.9754
Epoch 141/200
18458/18458 [==============================] - 3s 143us/sample - loss: 0.0845 - accuracy: 0.9732 - precision: 0.9707 - recall: 0.9137 - val_loss: 0.0677 - val_accuracy: 0.9783 - val_precision: 0.9632 - val_recall: 0.9414
Epoch 142/200
18458/18458 [==============================] - 3s 137us/sample - loss: 0.0894 - accuracy: 0.9720 - precision: 0.9707 - recall: 0.9084 - val_loss: 0.1276 - val_accuracy: 0.9614 - val_precision: 0.8691 - val_recall: 0.9792
Epoch 143/200
18458/18458 [==============================] - 3s 137us/sample - loss: 0.0876 - accuracy: 0.9717 - precision: 0.9675 - recall: 0.9105 - val_loss: 0.1315 - val_accuracy: 0.9575 - val_precision: 0.8598 - val_recall: 0.9735
Epoch 144/200
18458/18458 [==============================] - 2s 134us/sample - loss: 0.0983 - accuracy: 0.9679 - precision: 0.9683 - recall: 0.8930 - val_loss: 0.0673 - val_accuracy: 0.9805 - val_precision: 0.9583 - val_recall: 0.9565
Epoch 145/200
18458/18458 [==============================] - 2s 131us/sample - loss: 0.0851 - accuracy: 0.9726 - precision: 0.9692 - recall: 0.9128 - val_loss: 0.0735 - val_accuracy: 0.9762 - val_precision: 0.9309 - val_recall: 0.9679
Epoch 146/200
18458/18458 [==============================] - 2s 131us/sample - loss: 0.0843 - accuracy: 0.9733 - precision: 0.9707 - recall: 0.9144 - val_loss: 0.0745 - val_accuracy: 0.9762 - val_precision: 0.9309 - val_recall: 0.9679
Epoch 147/200
18458/18458 [==============================] - 2s 131us/sample - loss: 0.0854 - accuracy: 0.9731 - precision: 0.9718 - recall: 0.9121 - val_loss: 0.0767 - val_accuracy: 0.9740 - val_precision: 0.9718 - val_recall: 0.9130
Epoch 148/200
18458/18458 [==============================] - 2s 131us/sample - loss: 0.0862 - accuracy: 0.9727 - precision: 0.9694 - recall: 0.9130 - val_loss: 0.0895 - val_accuracy: 0.9705 - val_precision: 0.9094 - val_recall: 0.9679
Epoch 149/200
18458/18458 [==============================] - 2s 133us/sample - loss: 0.0892 - accuracy: 0.9712 - precision: 0.9683 - recall: 0.9072 - val_loss: 0.0793 - val_accuracy: 0.9779 - val_precision: 0.9377 - val_recall: 0.9679
Epoch 150/200
18458/18458 [==============================] - 3s 136us/sample - loss: 0.0947 - accuracy: 0.9705 - precision: 0.9696 - recall: 0.9031 - val_loss: 0.1545 - val_accuracy: 0.9536 - val_precision: 0.8425 - val_recall: 0.9811
Epoch 151/200
18458/18458 [==============================] - 3s 137us/sample - loss: 0.0906 - accuracy: 0.9716 - precision: 0.9681 - recall: 0.9091 - val_loss: 0.0634 - val_accuracy: 0.9788 - val_precision: 0.9858 - val_recall: 0.9206
Epoch 152/200
18458/18458 [==============================] - 3s 136us/sample - loss: 0.0887 - accuracy: 0.9726 - precision: 0.9720 - recall: 0.9098 - val_loss: 0.1612 - val_accuracy: 0.9502 - val_precision: 0.8339 - val_recall: 0.9773
Epoch 153/200
18458/18458 [==============================] - 2s 134us/sample - loss: 0.0977 - accuracy: 0.9687 - precision: 0.9663 - recall: 0.8983 - val_loss: 0.1101 - val_accuracy: 0.9645 - val_precision: 0.8807 - val_recall: 0.9773
Epoch 154/200
18458/18458 [==============================] - 2s 131us/sample - loss: 0.0745 - accuracy: 0.9771 - precision: 0.9750 - recall: 0.9263 - val_loss: 0.1646 - val_accuracy: 0.9571 - val_precision: 0.8525 - val_recall: 0.9830
Epoch 155/200
18458/18458 [==============================] - 3s 139us/sample - loss: 0.0822 - accuracy: 0.9737 - precision: 0.9735 - recall: 0.9130 - val_loss: 0.1084 - val_accuracy: 0.9623 - val_precision: 0.8877 - val_recall: 0.9565
Epoch 156/200
18458/18458 [==============================] - 2s 133us/sample - loss: 0.0830 - accuracy: 0.9721 - precision: 0.9664 - recall: 0.9132 - val_loss: 0.0739 - val_accuracy: 0.9766 - val_precision: 0.9326 - val_recall: 0.9679
Epoch 157/200
18458/18458 [==============================] - 3s 136us/sample - loss: 0.0809 - accuracy: 0.9742 - precision: 0.9736 - recall: 0.9153 - val_loss: 0.1272 - val_accuracy: 0.9692 - val_precision: 0.8976 - val_recall: 0.9773
Epoch 158/200
18458/18458 [==============================] - 2s 134us/sample - loss: 0.0957 - accuracy: 0.9708 - precision: 0.9685 - recall: 0.9054 - val_loss: 0.0702 - val_accuracy: 0.9801 - val_precision: 0.9464 - val_recall: 0.9679
Epoch 159/200
18458/18458 [==============================] - 2s 135us/sample - loss: 0.0760 - accuracy: 0.9756 - precision: 0.9739 - recall: 0.9208 - val_loss: 0.1130 - val_accuracy: 0.9736 - val_precision: 0.9224 - val_recall: 0.9660
Epoch 160/200
18458/18458 [==============================] - 2s 133us/sample - loss: 0.0873 - accuracy: 0.9726 - precision: 0.9722 - recall: 0.9098 - val_loss: 0.1625 - val_accuracy: 0.9484 - val_precision: 0.8213 - val_recall: 0.9905
Epoch 161/200
18458/18458 [==============================] - 2s 130us/sample - loss: 0.0889 - accuracy: 0.9708 - precision: 0.9696 - recall: 0.9042 - val_loss: 0.1490 - val_accuracy: 0.9510 - val_precision: 0.8344 - val_recall: 0.9811
Epoch 162/200
18458/18458 [==============================] - 2s 129us/sample - loss: 0.0771 - accuracy: 0.9758 - precision: 0.9735 - recall: 0.9222 - val_loss: 0.0893 - val_accuracy: 0.9740 - val_precision: 0.9180 - val_recall: 0.9735
Epoch 163/200
18458/18458 [==============================] - 2s 127us/sample - loss: 0.0778 - accuracy: 0.9752 - precision: 0.9725 - recall: 0.9208 - val_loss: 0.1083 - val_accuracy: 0.9645 - val_precision: 0.8834 - val_recall: 0.9735
Epoch 164/200
18458/18458 [==============================] - 2s 128us/sample - loss: 0.0917 - accuracy: 0.9722 - precision: 0.9738 - recall: 0.9061 - val_loss: 0.1728 - val_accuracy: 0.9410 - val_precision: 0.8066 - val_recall: 0.9773
Epoch 165/200
18458/18458 [==============================] - 2s 130us/sample - loss: 0.0893 - accuracy: 0.9721 - precision: 0.9689 - recall: 0.9107 - val_loss: 0.0724 - val_accuracy: 0.9805 - val_precision: 0.9465 - val_recall: 0.9698
Epoch 166/200
18458/18458 [==============================] - 2s 132us/sample - loss: 0.0803 - accuracy: 0.9741 - precision: 0.9719 - recall: 0.9164 - val_loss: 0.0907 - val_accuracy: 0.9701 - val_precision: 0.9244 - val_recall: 0.9471
Epoch 167/200
18458/18458 [==============================] - 2s 135us/sample - loss: 0.0843 - accuracy: 0.9752 - precision: 0.9744 - recall: 0.9187 - val_loss: 0.0675 - val_accuracy: 0.9805 - val_precision: 0.9515 - val_recall: 0.9641
Epoch 168/200
18458/18458 [==============================] - 2s 134us/sample - loss: 0.0885 - accuracy: 0.9733 - precision: 0.9723 - recall: 0.9128 - val_loss: 0.2243 - val_accuracy: 0.9207 - val_precision: 0.7464 - val_recall: 0.9905
Epoch 169/200
18458/18458 [==============================] - 2s 133us/sample - loss: 0.0760 - accuracy: 0.9772 - precision: 0.9778 - recall: 0.9240 - val_loss: 0.1398 - val_accuracy: 0.9545 - val_precision: 0.8464 - val_recall: 0.9792
Epoch 170/200
18458/18458 [==============================] - 3s 137us/sample - loss: 0.0936 - accuracy: 0.9717 - precision: 0.9716 - recall: 0.9063 - val_loss: 0.1020 - val_accuracy: 0.9649 - val_precision: 0.9870 - val_recall: 0.8582
Epoch 171/200
18458/18458 [==============================] - 2s 135us/sample - loss: 0.0839 - accuracy: 0.9727 - precision: 0.9724 - recall: 0.9098 - val_loss: 0.0609 - val_accuracy: 0.9818 - val_precision: 0.9728 - val_recall: 0.9471
Epoch 172/200
18458/18458 [==============================] - 2s 131us/sample - loss: 0.0874 - accuracy: 0.9721 - precision: 0.9717 - recall: 0.9079 - val_loss: 0.0986 - val_accuracy: 0.9684 - val_precision: 0.8972 - val_recall: 0.9735
Epoch 173/200
18458/18458 [==============================] - 2s 133us/sample - loss: 0.0808 - accuracy: 0.9742 - precision: 0.9724 - recall: 0.9164 - val_loss: 0.0894 - val_accuracy: 0.9727 - val_precision: 0.9146 - val_recall: 0.9716
Epoch 174/200
18458/18458 [==============================] - 3s 137us/sample - loss: 0.0875 - accuracy: 0.9709 - precision: 0.9685 - recall: 0.9058 - val_loss: 0.1509 - val_accuracy: 0.9471 - val_precision: 0.9880 - val_recall: 0.7788
Epoch 175/200
18458/18458 [==============================] - 3s 137us/sample - loss: 0.0845 - accuracy: 0.9733 - precision: 0.9739 - recall: 0.9111 - val_loss: 0.1013 - val_accuracy: 0.9666 - val_precision: 0.8897 - val_recall: 0.9754
Epoch 176/200
18458/18458 [==============================] - 3s 137us/sample - loss: 0.0906 - accuracy: 0.9718 - precision: 0.9721 - recall: 0.9063 - val_loss: 0.0845 - val_accuracy: 0.9762 - val_precision: 0.9293 - val_recall: 0.9698
Epoch 177/200
18458/18458 [==============================] - 2s 133us/sample - loss: 0.0943 - accuracy: 0.9703 - precision: 0.9691 - recall: 0.9026 - val_loss: 0.1231 - val_accuracy: 0.9580 - val_precision: 0.8636 - val_recall: 0.9698
Epoch 178/200
18458/18458 [==============================] - 2s 127us/sample - loss: 0.0820 - accuracy: 0.9752 - precision: 0.9741 - recall: 0.9190 - val_loss: 0.0791 - val_accuracy: 0.9731 - val_precision: 0.9238 - val_recall: 0.9622
Epoch 179/200
18458/18458 [==============================] - 2s 128us/sample - loss: 0.0810 - accuracy: 0.9746 - precision: 0.9736 - recall: 0.9171 - val_loss: 0.0765 - val_accuracy: 0.9757 - val_precision: 0.9308 - val_recall: 0.9660
Epoch 180/200
18458/18458 [==============================] - 2s 130us/sample - loss: 0.0872 - accuracy: 0.9720 - precision: 0.9705 - recall: 0.9086 - val_loss: 0.0805 - val_accuracy: 0.9753 - val_precision: 0.9245 - val_recall: 0.9716
Epoch 181/200
18458/18458 [==============================] - 2s 130us/sample - loss: 0.0781 - accuracy: 0.9755 - precision: 0.9739 - recall: 0.9206 - val_loss: 0.1136 - val_accuracy: 0.9606 - val_precision: 0.8712 - val_recall: 0.9716
Epoch 182/200
18458/18458 [==============================] - 2s 133us/sample - loss: 0.0909 - accuracy: 0.9705 - precision: 0.9708 - recall: 0.9017 - val_loss: 0.0902 - val_accuracy: 0.9718 - val_precision: 0.9143 - val_recall: 0.9679
Epoch 183/200
18458/18458 [==============================] - 3s 136us/sample - loss: 0.0750 - accuracy: 0.9774 - precision: 0.9776 - recall: 0.9252 - val_loss: 0.0919 - val_accuracy: 0.9701 - val_precision: 0.8993 - val_recall: 0.9792
Epoch 184/200
18458/18458 [==============================] - 2s 135us/sample - loss: 0.0709 - accuracy: 0.9789 - precision: 0.9778 - recall: 0.9316 - val_loss: 0.1111 - val_accuracy: 0.9640 - val_precision: 0.8805 - val_recall: 0.9754
Epoch 185/200
18458/18458 [==============================] - 3s 136us/sample - loss: 0.0779 - accuracy: 0.9749 - precision: 0.9741 - recall: 0.9176 - val_loss: 0.0660 - val_accuracy: 0.9796 - val_precision: 0.9382 - val_recall: 0.9754
Epoch 186/200
18458/18458 [==============================] - 2s 135us/sample - loss: 0.0750 - accuracy: 0.9761 - precision: 0.9735 - recall: 0.9233 - val_loss: 0.1257 - val_accuracy: 0.9610 - val_precision: 0.8714 - val_recall: 0.9735
Epoch 187/200
18458/18458 [==============================] - 2s 135us/sample - loss: 0.0818 - accuracy: 0.9738 - precision: 0.9719 - recall: 0.9153 - val_loss: 0.0921 - val_accuracy: 0.9675 - val_precision: 0.8982 - val_recall: 0.9679
Epoch 188/200
18458/18458 [==============================] - 3s 140us/sample - loss: 0.0838 - accuracy: 0.9730 - precision: 0.9711 - recall: 0.9123 - val_loss: 0.0624 - val_accuracy: 0.9840 - val_precision: 0.9677 - val_recall: 0.9622
Epoch 189/200
18458/18458 [==============================] - 3s 136us/sample - loss: 0.0829 - accuracy: 0.9742 - precision: 0.9729 - recall: 0.9160 - val_loss: 0.0748 - val_accuracy: 0.9766 - val_precision: 0.9342 - val_recall: 0.9660
Epoch 190/200
18458/18458 [==============================] - 3s 153us/sample - loss: 0.0881 - accuracy: 0.9717 - precision: 0.9700 - recall: 0.9079 - val_loss: 0.0644 - val_accuracy: 0.9801 - val_precision: 0.9464 - val_recall: 0.9679
Epoch 191/200
18458/18458 [==============================] - 3s 150us/sample - loss: 0.0774 - accuracy: 0.9759 - precision: 0.9756 - recall: 0.9208 - val_loss: 0.0579 - val_accuracy: 0.9840 - val_precision: 0.9624 - val_recall: 0.9679
Epoch 192/200
18458/18458 [==============================] - 3s 141us/sample - loss: 0.0717 - accuracy: 0.9779 - precision: 0.9742 - recall: 0.9307 - val_loss: 0.1076 - val_accuracy: 0.9640 - val_precision: 0.8845 - val_recall: 0.9698
Epoch 193/200
18458/18458 [==============================] - 3s 141us/sample - loss: 0.0916 - accuracy: 0.9706 - precision: 0.9692 - recall: 0.9040 - val_loss: 0.0836 - val_accuracy: 0.9731 - val_precision: 0.9162 - val_recall: 0.9716
Epoch 194/200
18458/18458 [==============================] - 3s 142us/sample - loss: 0.0653 - accuracy: 0.9797 - precision: 0.9806 - recall: 0.9321 - val_loss: 0.0641 - val_accuracy: 0.9805 - val_precision: 0.9449 - val_recall: 0.9716
Epoch 195/200
18458/18458 [==============================] - 3s 140us/sample - loss: 0.0856 - accuracy: 0.9733 - precision: 0.9709 - recall: 0.9139 - val_loss: 0.1037 - val_accuracy: 0.9662 - val_precision: 0.8881 - val_recall: 0.9754
Epoch 196/200
18458/18458 [==============================] - 3s 146us/sample - loss: 0.0813 - accuracy: 0.9735 - precision: 0.9695 - recall: 0.9162 - val_loss: 0.1329 - val_accuracy: 0.9601 - val_precision: 0.8660 - val_recall: 0.9773
Epoch 197/200
18458/18458 [==============================] - 3s 139us/sample - loss: 0.0766 - accuracy: 0.9764 - precision: 0.9766 - recall: 0.9217 - val_loss: 0.0815 - val_accuracy: 0.9705 - val_precision: 0.9080 - val_recall: 0.9698
Epoch 198/200
18458/18458 [==============================] - 2s 135us/sample - loss: 0.0770 - accuracy: 0.9752 - precision: 0.9721 - recall: 0.9210 - val_loss: 0.0955 - val_accuracy: 0.9697 - val_precision: 0.9033 - val_recall: 0.9716
Epoch 199/200
18458/18458 [==============================] - 2s 135us/sample - loss: 0.0745 - accuracy: 0.9766 - precision: 0.9734 - recall: 0.9259 - val_loss: 0.0863 - val_accuracy: 0.9688 - val_precision: 0.9059 - val_recall: 0.9641
Epoch 200/200
18458/18458 [==============================] - 3s 149us/sample - loss: 0.0731 - accuracy: 0.9768 - precision: 0.9750 - recall: 0.9250 - val_loss: 0.0722 - val_accuracy: 0.9740 - val_precision: 0.9241 - val_recall: 0.9660
We’ve got a trained CNN! What can we learn from it? Behind the scenes, \(\texttt{stella}\) creates a table of the history output by each model run. What’s in your history depends on your metrics. So, for example, the default metrics are ‘accuracy’, ‘precision’, and ‘recall’, so in our \(\texttt{cnn.history_table}\) we see columns for each of these values from the training set as well as from the validation set (the columns beginning with ‘val_’).
[30]:
cnn.history_table
[30]:
loss_s0002 | accuracy_s0002 | precision_s0002 | recall_s0002 | val_loss_s0002 | val_accuracy_s0002 | val_precision_s0002 | val_recall_s0002 |
---|---|---|---|---|---|---|---|
float64 | float32 | float32 | float32 | float64 | float32 | float32 | float32 |
0.5494471863194733 | 0.7645465 | 0.25 | 0.00023020258 | 0.5289232612599218 | 0.7706979 | 0.0 | 0.0 |
0.5323696360742817 | 0.7646549 | 0.0 | 0.0 | 0.4919142103073759 | 0.7706979 | 0.0 | 0.0 |
0.4736867796588091 | 0.7862715 | 0.97613364 | 0.09415285 | 0.38631349878234683 | 0.846554 | 0.99435025 | 0.3327032 |
0.36041638068794263 | 0.8620111 | 0.96530294 | 0.4290976 | 0.3165891189685259 | 0.86432594 | 0.9864865 | 0.41398865 |
0.3119629817292367 | 0.8820024 | 0.9577346 | 0.52163905 | 0.24189111077532294 | 0.9016038 | 0.9808917 | 0.5822306 |
0.29376881588111137 | 0.89478815 | 0.9474665 | 0.5854052 | 0.2646928105873105 | 0.8881664 | 0.98916966 | 0.5179584 |
0.2628104354419692 | 0.90551525 | 0.9513889 | 0.63075507 | 0.21784497176768927 | 0.9137408 | 0.97965115 | 0.63705105 |
0.2714826945868974 | 0.9031314 | 0.94221455 | 0.6268416 | 0.2428721376813021 | 0.9068054 | 0.9815951 | 0.60491496 |
0.2567230729437272 | 0.9083324 | 0.9310793 | 0.6593002 | 0.2139414006825593 | 0.92717814 | 0.9640103 | 0.7088847 |
0.244666275207342 | 0.914021 | 0.9229825 | 0.69244933 | 0.2233845726928 | 0.92717814 | 0.9592875 | 0.7126654 |
... | ... | ... | ... | ... | ... | ... | ... |
0.0773628002437499 | 0.9759454 | 0.9756098 | 0.92081034 | 0.05785705019991585 | 0.9839619 | 0.96240604 | 0.9678639 |
0.07167464291305722 | 0.97789574 | 0.9742169 | 0.930709 | 0.10761617743509075 | 0.9640225 | 0.88448274 | 0.9697543 |
0.09162614602982669 | 0.970636 | 0.969151 | 0.9040055 | 0.08356246228180875 | 0.9731253 | 0.916221 | 0.97164464 |
0.06531601481911439 | 0.9796836 | 0.98062485 | 0.9320902 | 0.0641237216293941 | 0.98049414 | 0.94485295 | 0.97164464 |
0.08562272742490738 | 0.97329074 | 0.97089756 | 0.91390425 | 0.1036811023240075 | 0.96618986 | 0.8881239 | 0.9754253 |
0.08131803582874625 | 0.9735074 | 0.96954936 | 0.91620624 | 0.13287109423365798 | 0.9601214 | 0.86599666 | 0.97731566 |
0.07659747941817684 | 0.9763788 | 0.9765854 | 0.9217311 | 0.081510869220164 | 0.9705245 | 0.9079646 | 0.9697543 |
0.07699505046901278 | 0.9751869 | 0.97206026 | 0.92104053 | 0.09550375936090248 | 0.96965754 | 0.9033392 | 0.97164464 |
0.07447957267920366 | 0.9765955 | 0.9733785 | 0.92587477 | 0.0863423299417384 | 0.96879065 | 0.90586144 | 0.9640832 |
0.07305251633495526 | 0.97675806 | 0.97500604 | 0.92495394 | 0.0722398692051673 | 0.97399217 | 0.9240506 | 0.96597356 |
It also keeps track of the ground truth (gt) values from your validation set flares and no-flares and what each model predicts. This table includes the TIC ID, gt label (0 = no flare; 1 = flare), tpeak (the time of the flare from the catalog), and, depending on the number of models you run, columns of the predicted labels. Each column keeps track of the random seed used to run that model.
[31]:
cnn.val_pred_table
[31]:
tic | gt | tpeak | pred_s0002 |
---|---|---|---|
float64 | int64 | float64 | float32 |
55269690.0 | 0 | 1332.7376590932145 | 0.0053598885 |
201795667.0 | 1 | 1373.0537959924561 | 1.0 |
80453023.0 | 0 | 1374.3399511708512 | 0.00066155713 |
161172848.0 | 0 | 1343.1752241130807 | 0.020634037 |
231122278.0 | 0 | 1340.0770763736205 | 0.020502886 |
25132694.0 | 0 | 1355.0857187085387 | 0.009274018 |
31740375.0 | 1 | 1351.193163007814 | 0.99998176 |
31852565.0 | 0 | 1332.3193510129825 | 0.016599169 |
220557560.0 | 1 | 1345.0766190177035 | 0.99853826 |
31740375.0 | 0 | 1380.7584138286325 | 9.603994e-05 |
... | ... | ... | ... |
5727213.0 | 0 | 1377.555473552861 | 0.0006570381 |
25132999.0 | 0 | 1375.6855870175875 | 0.057495333 |
176955267.0 | 1 | 1335.2901855122575 | 1.0 |
231910796.0 | 0 | 1365.9642105949472 | 0.0011209704 |
231831315.0 | 1 | 1370.6859209103193 | 1.0 |
33837062.0 | 0 | 1372.1118069837337 | 2.2111965e-06 |
231017428.0 | 1 | 1361.1166294240243 | 0.999871 |
114794572.0 | 0 | 1357.4690767472318 | 0.012727063 |
139996019.0 | 0 | 1336.5018758695448 | 0.014939568 |
118327563.0 | 0 | 1369.8558114699342 | 0.45524606 |
We can visualize it this way, by plotting the time of flare peak versus the prediction of being a flare as determined by the CNN. This can be thought of as a probability. The points are colored by the ground truth of if that point is a flare or not as labeled in the initial catalog.
[32]:
plt.figure(figsize=(10,4))
plt.scatter(cnn.val_pred_table['tpeak'], cnn.val_pred_table['pred_s0002'],
c=cnn.val_pred_table['gt'], vmin=0, vmax=1)
plt.xlabel('Tpeak [BJD - 2457000]')
plt.ylabel('Probability of Flare')
plt.colorbar(label='Ground Truth');
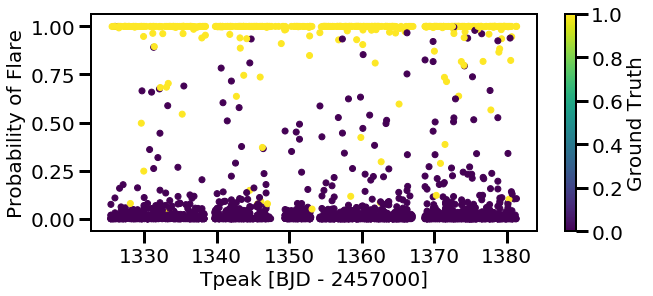
Most of the points with high probabilities are actually flares (ground truth = 1), which is great! The CNN is not perfect, but here is where ensembling a bunch of different models with different initial random seeds. By averaging across models, you can beat down the number of false positives (no flares with high probabilities) and false negatives (flares with low probabilities).
1.3 Evaluating your Model¶
How do you know if the model you created and trained is good? There are a few different metrics you can look at. The first is looking at your loss and accuracy histories. Here are some features you should look for:
If your training and validation loss smoothly decline and flatten out at a low number, that’s good!
If your validation loss traces your training loss, that’s good!
If your validation loss starts to increase, your model is beginning to overfit. Rerun the model for fewer epochs and this should solve the issue.
[33]:
plt.figure(figsize=(7,4))
plt.plot(cnn.history_table['loss_s0002'], 'k', label='Training', lw=3)
plt.plot(cnn.history_table['val_loss_s0002'], 'darkorange', label='Validation', lw=3)
plt.xlabel('Epochs')
plt.ylabel('Loss')
plt.legend();
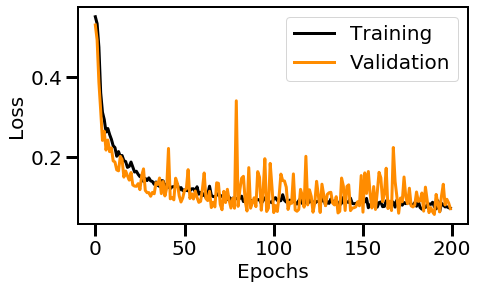
Some of the same rules as above apply here:
If your accuracy increases smoothly and levels out at a high number, that’s good! It means your model is at that leveling value % accuracy.
[35]:
plt.figure(figsize=(7,4))
plt.plot(cnn.history_table['accuracy_s0002'], 'k', label='Training', lw=3)
plt.plot(cnn.history_table['val_accuracy_s0002'], 'darkorange', label='Validation', lw=3)
plt.xlabel('Epochs')
plt.ylabel('Accuracy')
plt.legend();
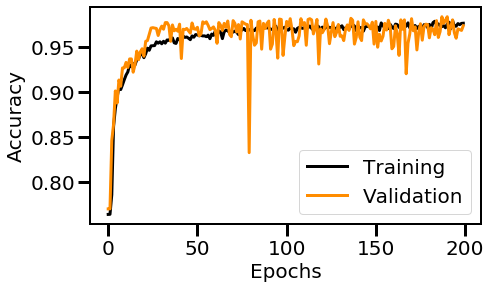
1.4 Predicting Flares in your Data¶
The function to predict on light curves takes care of the pre-processing for you. All you have to do is pass in an array of times, fluxes, and flux errors. So load in your files in whatever manner you like. For this example, we’ll call a light curve using lightkurve.
[37]:
#### create a lightkurve for a two minute target here for the example
from lightkurve.search import search_lightcurvefile
lc = search_lightcurvefile(target='tic62124646', mission='TESS')
lc = lc.download().PDCSAP_FLUX
lc.plot()
//anaconda3/lib/python3.7/site-packages/lightkurve/lightcurvefile.py:47: LightkurveWarning: `LightCurveFile.header` is deprecated, please use `LightCurveFile.get_header()` instead.
LightkurveWarning)
[37]:
<matplotlib.axes._subplots.AxesSubplot at 0x1474df978>
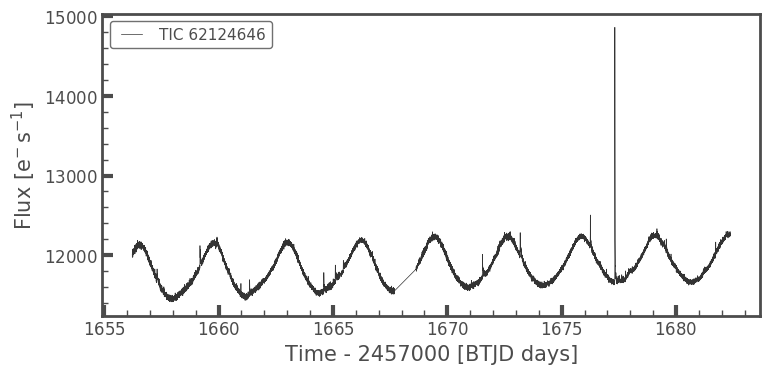
Now we can use the model we saved to predict flares on new light curves! This is where it becomes important to keep track of your models and your output directory. To be extra sure you know what model you’re using, in order to predict on new light curves you \(\textit{must}\) input the model filename.
[38]:
cnn.predict(modelname='/Users/arcticfox/Desktop/results/ensemble_s0002_i0050_b0.73.h5',
times=lc.time,
fluxes=lc.flux,
errs=lc.flux_err)
100%|██████████| 1/1 [00:00<00:00, 1.29it/s]
Et voila… Predictions!
[39]:
plt.figure(figsize=(14,4))
plt.scatter(cnn.predict_time[0], cnn.predict_flux[0],
c=cnn.predictions[0], vmin=0, vmax=1)
plt.colorbar(label='Probability of Flare')
plt.xlabel('Time [BJD-2457000]')
plt.ylabel('Normalized Flux')
plt.title('TIC {}'.format(lc.targetid));
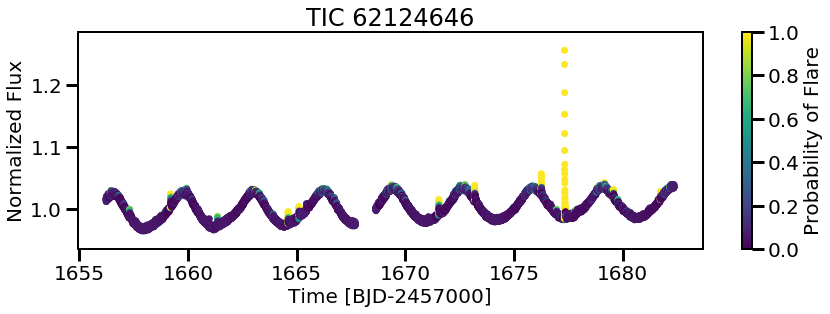